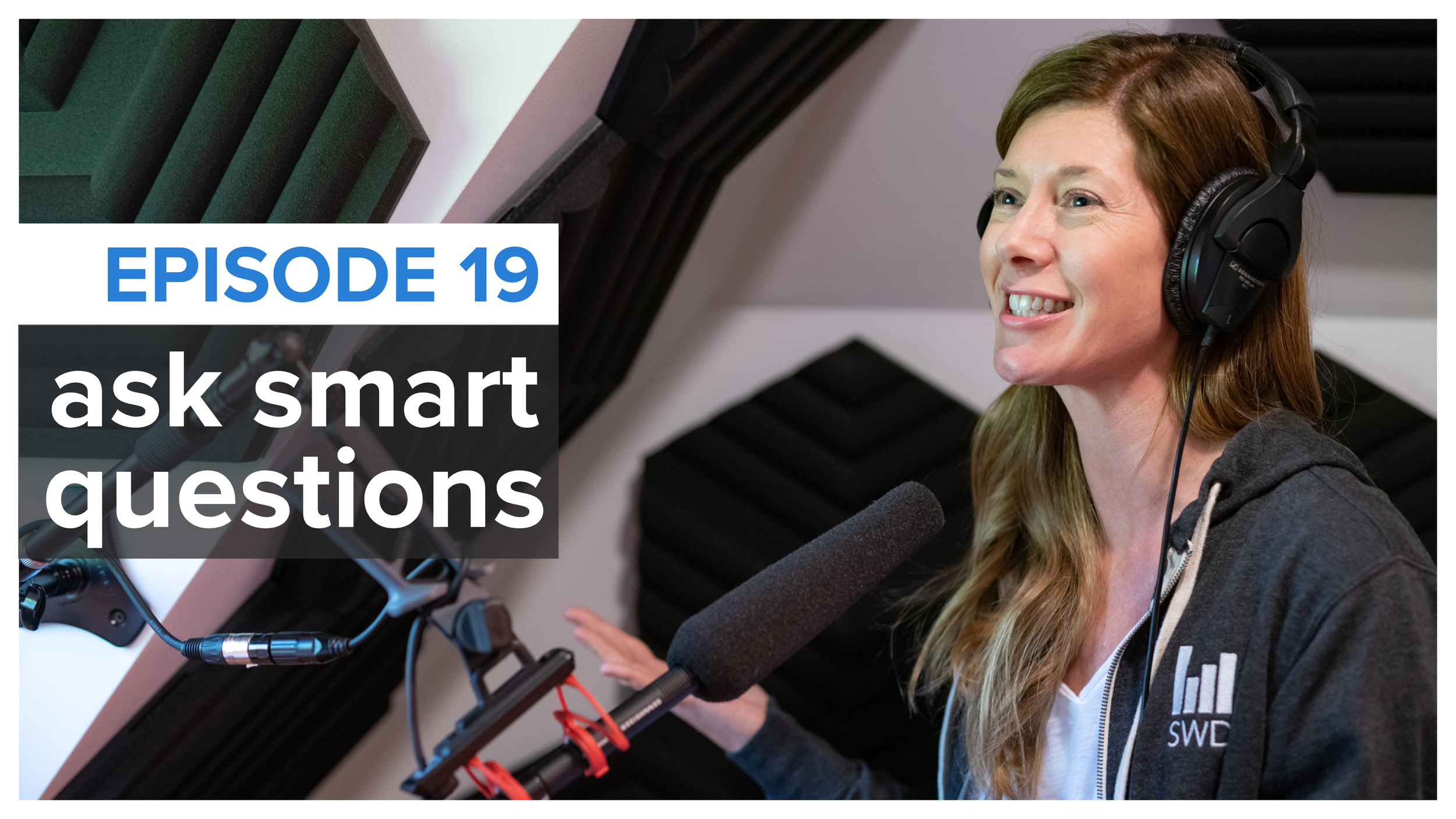
EPISODE 19
ask smart questions
Asking good questions can help improve our own and others’ work: both when exploring and explaining data. Tune in to hear Cole cover five smart queries to pose and answer during the analytical process and five more to consider when you are getting ready to explain data to others. Cole also answers listener questions on collecting requirements for good viz, what to do when asked to prove something with data that doesn’t pan out, and strategies for effectively presenting remotely.
RELATED LINKS
Register for a public workshop
Questions? email askcole@storytellingwithdata.com
Follow @storywithdata | share via #SWDpodcast
WE’D LOVE TO HEAR FROM YOU
Did you enjoy this episode? Do you have ideas for future episode guests or topics? Let us know!
Listen to the SWD podcast on your favorite platform
Subscribe in your favorite podcast platform to never miss an episode.
Like what you hear? Please rate & review. Thanks for listening!
TIMESTAMPS
00:32 | intro
03:10 | 5 questions to ask when exploring data
21:33 | 5 questions to ask when explaining data
30:51 | listener Q&A
TRANSCRIPT
Welcome to storytelling with data, the podcast where listeners around the world learn to be better storytellers and presenters with best selling author, speaker, and workshop guru Cole Nussbaumer Knaflic. We'll cover a wide range of topics that will help you effectively show and tell your data stories. So get ready to separate yourself from the mess of 3D exploding pie charts and deliver knockout presentations. And with that here's Cole.
[00:00:32]
“How do you figure out where to focus in the first place?” This is a question that is posed to me frequently. I spend a lot of time writing and teaching about how to communicate effectively with data. Certainly one important part of that process is knowing what exactly it is you want to communicate. One way to get there is to ask good questions. We’re going to talk about that today, but first, a quick personal anecdote.
My oldest child is 6. And he’s clever. I can remember when he was younger, he would pose very direct questions. For example: can I have a dessert? Now, that’s a yes or no question, right? Yes ends in delight and no in disappointment. No more conversation after that.
The next evolution in his way of asking questions was slightly better positioning, “Mom, if I finish dinner, can I have a dessert after?” So now he’s volunteering something that he knows I would like to see happen in order to try to make what he would like to happen more likely. But that’s still pretty direct. And remember, I said he’s clever.
So out of the blue the other day in the afternoon, he makes a statement to me: “Mom, you don’t like it when I’m sad.” No, of course not! “You like it when I’m happy, right?” Well, there’s only one way to answer that question: Of course dear, I love it when you’re happy—that makes me happy. And I totally should have seen this coming and you can perhaps anticipate where this is going. He says to me: “Well, it would make me so happy if I could have a dessert tonight. If I behave today and eat my dinner, could that be possible?”
Wow.
So I’m still not a fan of sugar, but I found that to be a pretty compelling build-up from a 6-year-old and certainly a much better way to frame the question!
Now, that’s not to say that we should do exactly that when analyzing data—framing our question to be more likely to get the answer we want—quite the opposite, in fact. Rather, this is to emphasize how being smart and robust in the way that we ask ourselves and others questions can help us frame our analytical work.
Let’s consider the question I began with: “How do you figure out where to focus in the first place?” Today, we’re going to talk about 5 questions you can ask yourself or others when exploring data, and 5 smart questions to ask as you prepare to explain that data to others.
[00:03:10]
So let's start talking about analyzing or exploring our data. One smart question to ask yourself is: is my summary statistic hiding something interesting? We often summarize data. We use averages or we combine things into groups and categories. This is important to do because data, when there's a lot of it, is sort of hard to comprehend. So by using these summary statistics or summary metrics, we can turn it into something in aggregate that's easier to talk about and we'll maybe see trends.
But there's also a dangerous side of this summarization. Let's talk about a couple of specific metrics and what we might want to look at underneath just to make sure we know our data well enough and aren't making bad conclusions.
Let's start with a common one, averages. Any time you're summarizing data in an average, you want to look at the underlying distribution, because when we are doing things with averages, we assume implicitly that the underlying distribution is normal; right, it has a bell-shaped curve. And that's not always the case. So you want to look at the distribution to see what's the spread? What is the range of values? Are there outliers that may be interesting or help you understand something better? Does the shape look like you expect—is it a big number and it is a normal distribution? Or is it skewed in some way that might make you cause how you summarize the data different?
This is particularly important when you are comparing averages. When you take two summary statistics and compare them to each other, you lose complete visibility to what those underlying distributions look like. They often will overlap in ways that could be interesting. The average might show a difference, but when you look at the underlying distributions, you may see that the difference either isn't exactly what you thought it was going to be or there might be something interesting in the overlap that causes you to talk about your data differently.
In some cases, you may find when you do this next level down and look at the underlying distribution, maybe the average isn't the best summary metric to use. There might be some sort of binning or categorization, or in some cases you might look at things by deciles or quartiles or different views that will allow you to see and potentially explain different nuances about the data. And other times, most of the time probably, actually, everything is fine. It is what you expect it is going to be, the underlying distributions are normal, the overlap is maybe minimal, and averages are a fine way to look at things. But anytime you find yourself summarizing in averages, be sure to take a look at the underlying distributions, make sure you're saying smart things and making good comparisons.
A couple other less common than average, but that I see enough to warrant talking about specifically, and there'll be probably other ways that you summarize data in your industry that you may be able to relate through these. One is percent favorable. This is one that's used often in survey analysis, where if you consider a Likert scale, for example, where you have five buckets of potential responses to a survey question. Someone can strongly disagree, they can disagree, they may have a neutral category, agree, and strongly agree. So one common way to summarize this across groups is percent favorable, which would be the proportion of people who selected, in this case, agree and strongly agree together out of anybody who completed the survey or everybody who completed that particular question if you're looking at a specific survey item.
What can happen here is when you're just looking at percent favorable, you miss other movement that maybe happening. Particularly interesting at times can be when you see people shifting from strongly agree to agree. Right, you lose that because you're bucketing those two together. You also miss seeing how you're neutral category is performing, and we'll talk about that a little bit more in the next stat that I talk about. But you can have neutral shifting into negative perceptions that you totally miss if you're just looking at percent favorable, and other movement between the categories that can be interesting or telling at times.
So like averages, with percent favorable, this doesn't mean that's not a good metric to use. Oftentimes, it is and it simplifies things in a way that allows us to compare across survey items and categories or across other categorical variables. But it's just a reminder to be looking at that next level underneath to make sure we aren't hiding anything interesting when we summarize in this way.
One more metric just to call out specifically is NPS, net promoter score. This is a metric that's used commonly in voice of customer analytics. And NPS, so this can be any product or it's used often with apps and such where your customers or your clients rate you on some sort of a scale, rate your service, rate your product. Sometimes it's a five-point scale similar to what we just talked about with the Likert scale, or sometimes a ten-point or a hundred-point scale. The scale itself doesn't matter. But what happens is we then bucket people or responses based on how they've answered that or what their numerical ranking is. Typically, the low categories are detractors, those are people not likely to recommend your service or your product or your business to others. There's the neutral category. And then there's the promoter category at the top. So these are the ones that are likely be talking positively about your brand or your product to others.
And so NPS, the net promoter score, is the proportion of promoters minus the proportion of detractors. So the higher number the better. Having NPS and the way it's consistently calculated means companies can compare themselves with other companies in their industry or their products or over time in ways that can be interesting.
The danger is: we miss what can potentially sometimes be some interesting underlying movement. Specifically, I've seen cases where you miss what's happening with the neutrals. Because you can actually have a case where your net promoter score, your NPS, is going up, which is a good thing, but where you have neutrals that are shifting both into the positive bucket—the promoter buckets—as well as into the detractor bucket.
I saw a case one time where there was this polarization that was happening pretty extremely over time that you missed when you just looked at NPS. This is just another case in point to say anytime you're summarizing data, just take a look at what's going on underneath to see if your summary stat might be hiding something interesting.
Let's move on to another question. Actually, this is another way that we often summarize data: time.
Question number two is what is the appropriate frequency with which to show my data? We have a lot of data these days, a lot of things that can collect data all the time, which means we have a lot of different ways to slice data over time and different frequencies within which we can look at our data: hourly, daily, weekly, monthly, quartering, yearly. There's always a question of what is going to be the appropriate time frame and frequency of time to aggregate my data. Because you can imagine when you look at daily data for something that has broader drivers underneath, the daily data or hourly data, this granular level can sometimes look really noisy, and so if you find yourself looking at a graph where there's up and down and up and down and up and down and it's hard to see what's happening, that can be a case where actually aggregating your data more can make sense. So if you're dealing with noisy data on a daily basis, try going to weekly. Or noisy weekly data, try going to monthly. Noisy monthly data, sometimes going to quarterly view can make sense. And what this allows you to sometimes see is where there are overarching trends that are actually harder to isolate and be able to identify in the more granular frequency views of the data.
Now, the opposite is also true. If you have a nice smooth line as you're looking at your monthly data, chop it up a little more and see if that reveals anything interesting. What does it look like if you move to weekly data? Are there interesting commonalities you see from the beginning of the month to the end of the month? Or are there interesting things that happen over weekends or on a particular day of the week? These are things that you miss entirely when you're looking at data that is aggregated on a monthly basis that can sometimes be interesting or telling depending on what you're trying to understand about your data.
On the topic of time and frequency, another way that can sometimes be interesting to look at temporal data or things to be aware of is when there's seasonality. So oftentimes we look at data over time, where we start with one month and we just go forward month by month by month, quarter by quarter by quarter, forward over time. Now, if there's any seasonality, it can sometimes be interesting to change your x-axis up. So rather than, let's say, months over time, you have months of the calendar year: January through December. And then what that allows you to do is you may have a line or a bar, depending on what you're looking at, for each year of data along that monthly path, because what that allows you to do is see what the year-over-year change or difference or shape looks like. In some cases you'll see the lines have similar shapes, which may point to there being some seasonality to the data that's important to understand or communicate. In other cases you may suspect that there is seasonality, that those shapes would look similar, and they don't, so that can sometimes help you isolate some interesting things. That's a little bit on the appropriate frequency. The meta point here is when you're looking at data over time, step back and think about what is the right amount of time. If you're seeing things stable or things noisy, try looking at it from a different frequency standpoint and see whether that reveals anything interesting.
Let's move to another question, another good one when you're analyzing data, which is how do things vary—or not—by category?
Any time we have data, we typically have a lot of different things that we can use to slice and dice it by; by region, by product line, by customer type, or part of the business. Cohort can sometimes be an interesting way to look at data, where you line things up by some point in time. It could be the first time someone downloads your product. Or it could be, if looking at employee analytics, it could be the day that someone starts at your organization; you can line people up by that beginning point in time and sometimes see some really interesting things.
You always want to ask yourself, so if you see something changing at an aggregate level, at a total level, like something company-wide, you want to understand is it happening everywhere or is it concentrated in one or a couple of places?—because that can lead you to prompt different sorts of actions as a result of that.
I can think of an analysis that we were doing recently for a client where the original was some bars and a data table and it was some projections looking forward about what markets were going to look like in different regions for this particular product. In the bar chart, it was a little tough to see what was going on. We could see some things going up and some things going down, and when you played with some different views of the data, what you actually saw was the initial forecast the team had done in aggregate—they had done two forecasts out over time—so the initial forecast was actually quite a lot lower than the new updated forecast. But then when you break it down by region, actually, that didn't pan out for most of the regions. It was one particular region, I think it was EMEA (Europe, Middle East, and Africa) in this case, it was actually driving everything that you saw in the total.
Now, you can imagine how, if you never do the split down to what's happening across regions, you may not know that information, and you run the risk of making a decision or doing something blanket everywhere without having this next level of insight to really understand the data and influence smarter decisions and actions.
When you see something happening in aggregate, ask yourself how do things vary across different categories? What are the normal categories used to slice and dice your data? Do that so that when you go back, you can say not only here's what's happening, but here's where it's happening more or less, or here's what may be driving what we're seeing in aggregate and, again, can be smarter and then the next steps you recommend as a result of that.
Another thing that we can do is ask ourselves where are things different from what we expect? When we're analyzing data, particularly if it's in an explorative capacity, where we don't have a specific question we're trying to answer, we're just looking at the data to try to see where something noteworthy might be going on, this is a good question to ask yourself. Where are things pretty much in line with what I expect and where are they not? Oftentimes, we'll look at a ton of data and in most of the cases, there's nothing interesting going on. It's this process of turning over rocks to see if eventually you find something interesting.
In our workshops, I'll often liken it to you're hunting for pearls in oysters where you may have to open up a hundred or several hundred oyster shells to find a pearl or two, the interesting things about the data. You still had to look at all those several hundred oyster shells to find them and that and that's part of the process, but we don't need to focus on where things are what we expect most of the time. Rather, it's more interesting to dive into where things are different from what we expect. And so sometimes we'll have really specific things like targets or goals or averages that we're comparing things to in order to be able to identify where things are different from what we expect them to be. Other times this will just be tacit knowledge and sort of turning the data one way and turning it another way and seeing what interesting things jump out. And it's where things are different from what we expect, that's where we can start to oftentimes form some of those interesting stories. Like, hey, that's different than we thought it would be. Now let's dig down to that next level and understand why. That's where you then come back to some of these other questions of how do things break down by category or how might we change the frequency that we're looking at things or maybe we need to go deeper than our summary statistic in order to really be able to understand what's going on here.
Let's talk about one more question on the exploratory side which is a very important one; what assumptions am I making? Be clear on when we are assuming something about our data or about the context that may not actually pan out that way. When we're working with data individually, that's sometimes hard to figure out because we do this tacitly sometimes, where we may not even realize we're making assumptions, and this is one great place, or one great reason, I should say, to talk through what you're doing with someone else who's less familiar and have them try to poke holes and have them help you identify where you're making assumptions. If you can identify your assumptions, then you can ask yourself how big of a deal is it if those assumptions are wrong? Sometimes it's not a big deal. Sometimes you might be off a little here or off a little there, but directionally, it's not going to impact anything. That's where we need to let people know about the assumptions, but we can be less up front with them.
In other cases, if you're making an assumption that turns out not to pan out in that way and that materially changes what you're going to be talking about or the observation or the direction you recommend, that is a bigger deal and that's where we need to be very up front and appropriately caveat everything that's going in so that people who are potentially making decisions based on what you're doing can take that into account.
All right. So that was five questions you can ask while you're analyzing data. After the break, we'll discuss questions you can ask when explaining that data to others.
[00:20:35]
October 2019 is going to be an exciting time for fans of storytelling with data. First, October 16th will be the last public workshop of the year. Join us live from Chicago for this full day, interactive session where you will learn the fundamentals of better data storytelling from Cole and the entire storytelling with data team. For those not able to travel to Chicago Illinois, worry not—for the first time ever, this 1-day workshop will be live streamed to those who register for this option. visit storytellingwithdata.com/ to learn more.
Next October 22nd marks the release of Cole’s 2nd book, storytelling with data: Let’s Practice! This one-of-a-kind immersive learning experience will help you learn or teach others to learn how to become a powerful data storyteller. Build confidence and credibility creating graphs that make sense and weave them into action-inspiring stories. Expanding upon the foundational lessons of SWD, Let’s Practice! delivers fresh content, a plethora of new examples, and over 100 hands-on exercises. Pre-order today on Amazon or from your favorite book retailer and be one of the first to get your copy of the soon-to-be bestseller, Let’s Practice!
[00:21:33]
Welcome back. So before the break we talked about five questions you can ask yourself when you're exploring data. Next I want to shift to explaining data. You've analyzed your data. You have something interesting you want to say about it. What questions can you ask yourself to help set you up for success when it comes to communicating that data to someone else?
First question: What level of polish is warranted?
I think people sometimes leave our workshops thinking oh, dear, this means I have to do this for every bit of data that I touch going forward. That is not at all the case. We want to be strategic about where and how we use our energy and our polishing. If you're presenting something to your colleagues and rough and dirty tool output is okay, then stick with rough and dirty output.
If you're communicating to senior leaders at your organization, that warrants a different level of polish. When you're presenting data—that's what they see, of everything that was done behind the scenes, the entire analytical process. So you want that bit that they can see to say good things. That happens indirectly, and it's through a lot of the small decisions we make about how to show our data: aligning things and graphing it in a smart way, which we'll talk about more, and really taking time to make sure that the takeaway you need your audience to know comes across clearly and easily. It's by being smart about when we take the time to polish and when we don't that you get adequate time when you need to to be able to make your data and your communications look really professional and put together. Be smart even within a given document about how you spend your energy and time. For something you know that's going to go in the appendix and maybe someone will look at it and maybe they don't, that, again, can be the rough and dirty. Put tables in the appendices. That's fine. But for the main content, the main story, that's where you want to take the time to polish and make what people see say good things about the overall process.
Question number 2: What biases might your audience have that will cause them to buy in or resist?
This is always something good to think through and is another place where grabbing a colleague or two and talking through this so that you can get other people's viewpoints can be really useful. Or if you're thinking: I don't know my audience, I don't know what biases they may have—talk to somebody who has communicated with them before or knows them better than you do. Or talk to people who are similar to your audience. Or just step back and think about what do you know about your audience? What motivates them? What biases can you anticipate they might have and how does that mean you can frame what you're going to talk through in a way that either brings that up so that you can address it or walks them through your logic so that they can get around it? This is one of those areas where any amount of time you can spend thinking about your audience and how you'll be communicating and work to set yourself up for success, that will be time well spent.
Let's move right on to another question, which is: What visual will show what I need?
There's no single answer to this. Well, actually, there is a single answer to this. The visual you need to use when you're explaining data is a visual that will help you explain that data to your audience. This often means stepping back and being clear about what is it I want to enable my audience to do with the data that I'm showing and then choose a graph type that's going to help facilitate that action. The best way to figure that out is to graph your data and then iterate and look at a few different views of the data. This both allows you to get to know your data a little better, but, also, you'll see how different views allow you to see different things more or less easily and can help you isolate one of these ah-ha! views for your audience where you'll be able to do other things to the graph to help them see what they need to see. If you're ever in doubt, this is another fantastic place to grab a colleague and show them what you've created and have them talk you through their thought process, what they pay attention to, what questions they have, what observations they make. It can be useful to see things through someone's eyes and see if they're seeing what you need them to see or if you might need to iterate.
Another question: How will I be presenting to my audience?
Are you there live talking through something? Do you have a screen between you and your audience, you're presenting virtually? We'll talk about that a little bit more when we get to listener Q&A. Are you sending something out that's going to be consumed on its own? Are you maybe putting together materials that someone else is going to be presenting?
Be thoughtful in each case when you're designing your explanatory communications. How is it going to be communicated? Because this will direct you to make different decisions about how you show the data. If you're sending something off that's going to be consumed on its own, people will be printing it or they'll be looking at it on their monitor in front of them, you can get away with higher density of information there.
Versus if you're live in a room, are you handing things out or are you presenting on the big screen? If you're presenting on the big screen, less already looks like more and people have lower tolerance for density of information on the big screen. Now, there are still tricks you can do to build things up, but generally you want to keep your slides sparse so that what you're showing on the screen isn't competing with the words that you're saying and that people can adequately pay attention, as they need to, to both at the same time, so when you're saying words, there's something on the screen that's reinforcing the words that you're saying or showing it in a picture. You can think about some of the strategies, like building things piece by piece, particularly if you're going to end up with something that feels complicated or heavy. If you're smart about how you position that and how you build up to it, you can keep something that's complicated or heavy from feeling intimidating to your audience, talk them through it piece by piece.
Another question on the explanatory side, and actually this is a good question to be asking throughout the process of analyzing your data, explaining your data, which is: Who can give me good feedback?
There's actually an exercise in my new book that walks through considerations when it comes to feedback. Actually, a number of exercises that are around the area of getting feedback in different parts of the process. You always want to consider what do you need when it comes to feedback? Is it better to talk to somebody who has context? Or might it be useful to talk to somebody without any context? Right, that can sometimes help you identify better words to use or other ways to explain things that may be more accessible depending on who your audience is. Do they need to have a background in statistics or can it be someone who's less technical? You'll get different viewpoints from these different people and gain varying insights into how you might change either how you show things or how you talk about things depending on your audience and the assumptions you can make about them.
All right. So one final Q. This is another one you should be asking yourself every time you're looking at data and analyzing data and explaining data, which is: What can I learn from each process that can inform my work going forward?
Every time you explore data, pause and think about what worked well? Where did I spend the right amount of time? Where did I maybe go down a little path than took a little longer than it should have? Maybe I should have cut that off sooner. What good questions did I ask? What questions might I ask in the future? How might I reframe things if I do this again a quarter from now or a year from now?
Also think about where you can be efficient. Efficiency both in how you explore your data, but also efficient in how you then explain that data to someone else. Going back to one of the questions we talked about, what's the right level of polish that you need and optimizing given that.
Also, were you able to use your data to inform the understanding that you sought or drive the action that you thought needed to happen? That can be a great question to reflect on to consider your relative level of success. Because you may have done an awesome analysis and presented it well, but if it's not driving the action that you need, then there's still something to revisit in that process or to get feedback on from others so that you can figure out going forward how do we not just do a great job when we're exploring job and explaining data, but how do we best use that data to drive smart actions and smart decisions?
So in summary, ask smart questions of yourself and others to drive robust analysis and communicate your data in an effective way.
[00:30:51]
Let’s shift next to listener Q&A. Alex writes: How do I collect requirements to create a powerful visualisation?
This is a broad question, so I’m going to constrain it a bit and assume it’s posed about visualizing data in a business setting. This is actually another case where asking smart questions is key. For me, making it about the visualization isn’t quite the right focus. Rather, it’s understanding what your audience or users are trying to solve for. If you can talk with them to learn more about them and their needs, that’s ideal. I often encourage those in analytical roles working with data to consider themselves consultants: it’s not your job to deliver data. It’s your job to understand what the people who need the data are trying to solve for so you can provide the right information. Often people don’t know exactly what they need, and so a little bit of back and forth - or sometimes a lot of back and forth - is needed to make that clear. Why do they need the data: what is it going to help them do? Do they want to use it to show they should keep doing something the same or that they should change something? Is there broader context to understand? Do they have expectations about what the data will show? Is it going to be an issue if that’s not the case? The more you can get to understand not only what your stakeholders want, but also why they want it, the better you can deliver on the need.
Then once you’ve isolated the specific data of interest, you want to consider what you want to enable your audience to do with that data and choose a graph that will make that clear. Finally, it’s not just about the data, but focusing attention and putting words around it to make the takeaway or story clear. The way to create a powerful visualization isn’t to make a sexy graph—it’s to visualize data that answers a question or provides insight that wasn’t previously known that your audience or stakeholders can do something useful with.
This next question was from a workshop participant last week: You talk about the case a lot where you’ve analyzed data and have something you want to say from it. But what do you do when it happens the other way around: you’re handed the “so what” and asked to collect data that supports it. Specifically, what should you do when the data doesn’t support the “so what” you’ve been handed?
This can be a challenging scenario, but I’m guessing one that many people can relate to. Of course in part it depends on who is asking. But in general, you can treat this as hypothesis testing, where you’ve been handed a hypothesis and now you have to test it out. When things play out as assumed, there’s no issue. The issue is—and the crux of the question posed—when that’s not the case. We had some good conversation in the workshop last week around this and a couple interesting solutions posed. One scenario we talked about is where maybe things used to be a certain way but then changed. So you could anchor the conversation in this place where things align - let’s say the APAC sales manager wants to show sales growth in APAC, you can start by showing the strong growth two years ago and then shift into the current story where that doesn’t play out. And even if it doesn’t play out in total - this is where coming back to our smart questioning from earlier can be useful - slice and dice it by the various things to see where it maybe does, and where it does not. This can shift the conversation in interesting ways, where no longer are you saying, you want us to show x and the data says y, but rather here IS where they data says x, but in these other places it does not.
Now of course it depends on who is asking, but in cases where you need to push back, be thoughtful and careful about how you do so. If there is someone influential who you can use as a buffer, do so. You certainly want to caveat appropriately—what you can use the data to say, what you cannot, or the limitations.
I’ve really just scratched the surface here. This is not a perfect answer—perhaps there isn’t a perfect answer. I’m curious whether those listening now might have ideas. Is this a situation you’ve dealt with? Any words of advice or tips for success? Email askcole@storytellingwithdata.com and if we get some good ideas, I’ll be sure to follow up with those in a future episode.
Janine asks: What should you do differently when presenting data in-person versus on-video? Any tips for encouraging high-engagement on a skype presentation?
My ideal scenario for presenting is in person—so certainly if you have a choice, opt for that. You get a different level of control and influence when you are live with people and can watch their facial responses, or see when people are tuning in or out, and pause or speed up and do other things like that as you read the room to set you up for success. Even when we’re in the room with others, it’s hard because there’s always the possibility of people picking up their phone or opening up their laptop. This is worse in a virtual environment, because you can basically assume this is happening: you are competing for attention with someone’s email when you present to them remotely. Still, there are some things you can do. Use verbal cues when you need someone in your audience or some segment of your audience to tune in. Andy: you’ve going to care about this next part because it involves resources from your team. Or Finance, we’re talking budget next, please tune back in.
Another strategy I’ve found can sometimes be useful: and we use this a lot when in person, too: is building your graph or story piece by piece. Rather than a full slide that someone can scan and then turn back to their inbox, build a graph one piece at a time. Start with the skeleton: just the axes and axis titles to talk your audience through what you’re going to show them. Then add another data point and narrate the relevant context. Then another and do the same. There’s action on the screen and people are curious what will come next, might be afraid of missing something, which can help keep their attention. Engagement is sometimes harder in virtual land, so if you’re presenting something where you need participation or discussion, see if you can seed that with a person or two ahead of time. Just something to get things going and help set the expectation for everyone that your audience aren’t passive consumers, they are meant to be following and participating.
More broadly, when designing data communications, always think ahead to how you’ll be presenting. Anticipate how you can set yourself up for success in that specific scenario. Any time you spend thinking about this and planning for this will be time well spent.
I think that’s a great point to end with. Big thanks to everyone who submitted questions. If you have a question, you can email it to askcole@storytellingwithdata.com.
...with that… be sure to follow @storywithdata on twitter and instagram. Also check out our new LinkedIn page all the great resources on the blog at storytellingwithdata.com. Thanks for listening!
Listen to the SWD podcast on your favorite platform
Subscribe in your favorite podcast platform to never miss an episode.
Like what you hear? Please rate & review. Thanks for listening!