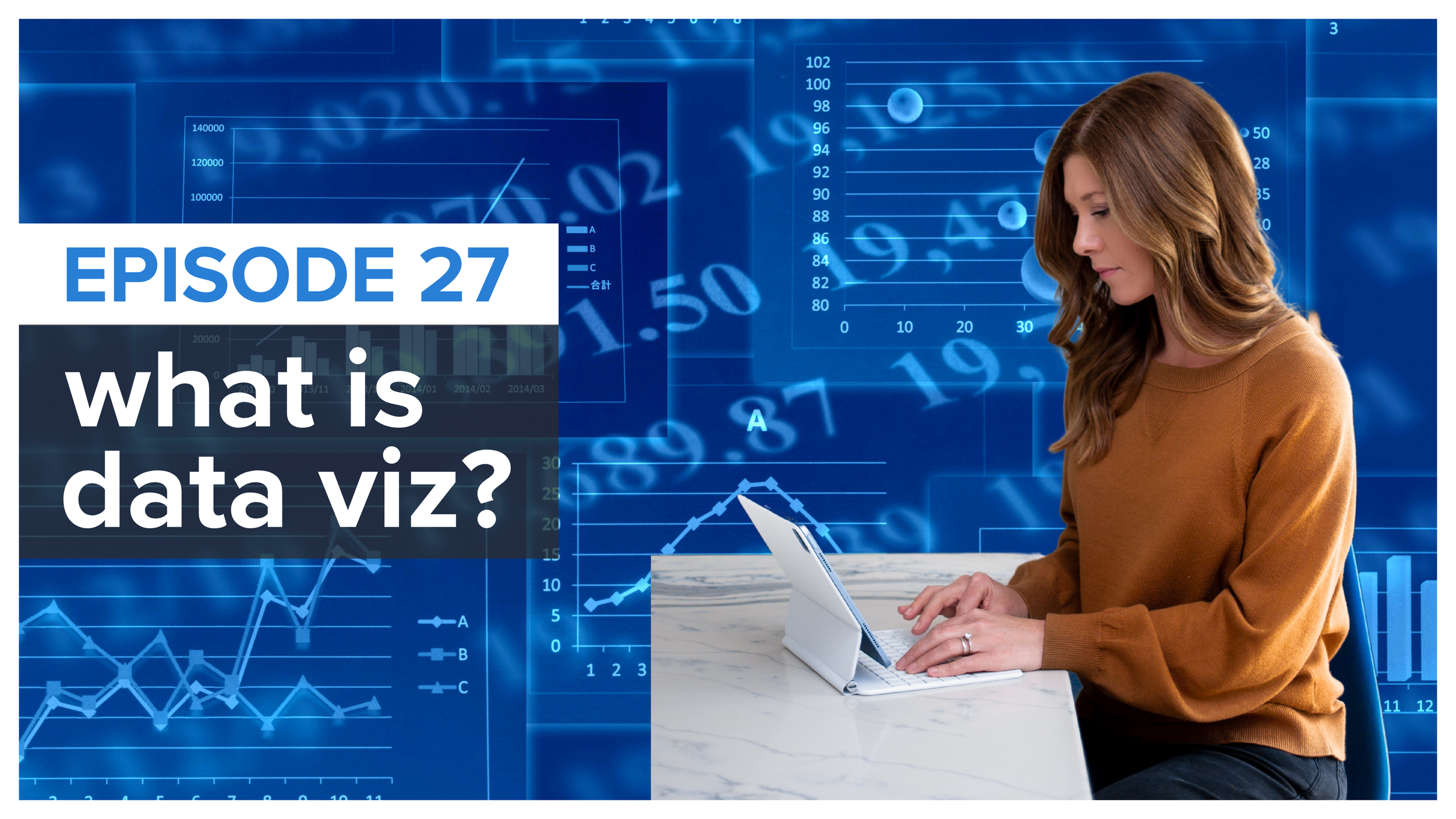
EPISODE 27
what is data visualization?
Why do we visualize data and what makes data visualization good? Tune in to listen to Cole lend her thoughts on these and related questions. She also answers listener questions about chronological versus lead-with-ending ordering for presentations, what to do when trying to show many data series in a line graph, and resources for communicating risk in a way that is easy to understand.
RELATED LINKS
Podcast: What makes a visualization memorable? (Data Stories)
Conversation: How to balance engaging & informing? (Charlie Hutcheson)
Visual: What makes a good data visualization? (David McCandless)
Medium post: Florence Nightingale is a design hero (RJ Andrews)
Exercise: Let’s draw!
SWD Let’s Practice! downloads (see solution 2.2 built in many tools)
Book: Data Points (Nathan Yau)
SWDchallenge: small multiples
Ask for feedback on your visual
Conversation: Resources for communicating risk
Workshops: 2020 public workshop schedule, custom workshops & webinars
Join the SWD community!
WE’D LOVE TO HEAR FROM YOU
Did you enjoy this episode? Do you have ideas for future episode guests or topics? Let us know!
Listen to the SWD podcast on your favorite platform
Subscribe in your favorite podcast platform to never miss an episode.
Like what you hear? Please rate & review. Thanks for listening!
TRANSCRIPT
Welcome to storytelling with data, the podcast where listeners around the world learn to be better storytellers and presenters with best selling author speaker and workshop guru Cole Nussbaumer Knaflic. We'll cover a wide range of topics that will help you effectively show and tell your data stories. So get ready to separate yourself from the mess of 3-D exploding pie charts and deliver knockout presentations. And with that here's Cole.
Hi, this is Cole! I am talking to you from a chilly Wisconsin today: the sun is out, but the ground remains covered with a thick white blanket of snow. They tell me winter will end here eventually but I’m not seeing it quite yet. I actually trudged through that snow earlier today, walking my kids to catch the bus for school. I have three young children. They are currently ages 7, 5, and the baby, who’s totally not a baby anymore, just turned 4.
I learn a lot from my kids and how they explore the world and try to make sense of things. One of the ways in which they do that is to ask a ton of questions. They used to be pretty basic ones, and for the little one (she’s the only girl), they still mainly are—things like, Mom, what’s your favorite color, or how old are you? But the boys’ questions (the older two) have become more difficult. Their line of questioning is more like: how old are you? How old are people when they die? When are you going to die? What happens when you die? Or, another popular progression these days goes something like: which is closer, the moon or the sun? How many moons can you fit in the sun? How big is space? How do we know? Where does it end? Or, sort of related, one of Dorian’s—he’s the middle one, who’s five—favorite topics currently is infinity. He’s trying to wrap his head around it. This is a complicated concept, right? I am still trying to wrap my head around it. Not a day goes by lately where he doesn’t ask me what the number before infinity is.
Honestly, these are much tougher inquiries than I anticipated would come from them at this point!
But let’s get back to this idea of posing questions to make sense of things. There’s a topic and some related questions I’ve seen lately that maybe will sound simple when I first pose it, but the answer is nuanced: what is data visualization?
So today, I’m going to share my thoughts on that and some related questions.
So let's start with that basic one: What is data viz? I think of it as turning numbers into pictures, and if I think back to my exposure to this, right, when I first experienced visualizing data directly, was in college. Nobody ever taught me what data viz was or how to visualize it, but I remember having to create some graphs in chemistry, I think it was, and at that point gave no thought to anything other than making the graph that I was asked to do.
For me, the turning point was really in my first job in banking; working in credit risk management, dealing with a ton of data, doing complicated stuff with it, and then needing to communicate that stuff to other people. That was where I started to see the power of taking numbers or aggregations of numbers and turning that into pictures that other people could see, that I could talk through or point to in a way that could explain something better, facilitate new understanding, allow us to create insights that we otherwise may not have been able to. So one result of visualizing data is that we can make things more broadly understandable and help people create a new understanding or a better understanding or a more informed understanding, and help them form new insights by virtue of being able to see and look at and visually explore something.
Another benefit of visualizing data is from a memorability standpoint. This is particularly powerful when we use both the pictures, the data visualization and words together with those pictures, where there are parts of our memory that are really fast at recalling images and pictures, which means if there's something interesting in the shape of the data and we're able to turn that into a graph that shows that and put words around it that describe that, all of those things work together to help improve the memorability of that point that we're trying to make and the data that we're showing.
Michelle Borkin at Northeastern University has done some really interesting research around memorability and data visualization. There's a podcast that she did, or an interview she did on the data stories podcast a year or two ago that I will make sure to link to in the show notes. That's definitely worth a listen.
So I'm going to parlay this what is data visualization question into a related one, which is why do we visualize data? And it turns out there's not a single answer to this question. We visualize data for a lot of different reasons. We might visualize data to analyze something or understand something better. We may visualize data to inform or help explain something to someone else. On a totally different end of the spectrum, in some cases we visualize data to entertain. Or we might do it for esthetic purposes, right; in the name of beauty, we find something that can become beautiful when we turn it into a data picture.
None of these purposes are better or worse. They're just different from each other. Some of the reasons we visualize data are behind the scenes, to understand what's going on, and some of those are more outward facing, right; to then try to explain that to someone else. Which means that who we're visualizing data for also becomes an interesting question in all of this. Are we visualizing for ourselves or are we visualizing it for someone else? Because the way in which we do so, the methods we employ, how we look at the data, the things we do to design it, may be very different as a function of who we're doing it for.
This brings me to the question that I've heard come up a few times lately and a question that comes up over time frequently enough, which is what makes data visualization good? What makes data visualization effective? The answer here probably won't surprise people, but it depends. Because for me, it goes back to why are we visualizing that data in the first place and what good looks like will change depending on that.
Charlie Hutcheson posed a question along these lines on Twitter recently and there was some interesting back and forth there, so I invited him to start a conversation on it in the storytelling with data community as well where we'd have a little bit more flexibility for a thoughtful conversation. His topic that he was trying to dig into was this balance between engaging and informing. He was noticing he lately has seen more novel approaches to visualizing data that may be eye-catching, but that when you dig in, it's actually not so easy to interpret what you're looking at. And so he's talking about that trade-off and when is it okay or is it okay and how upfront should the designer of the data be when they're doing something that may not be "best practice." Specifically, one of the sub questions he asked within his commentary is is it okay to engage an audience through an esthetic hook if it isn't subsequently easy to understand the underlying info. I think it's easy to say no, you know, that's not a good thing or yes, that is a good thing, but both of those answers can be correct in a given situation. So what good looks like is going to be dependent on the context always.
David McCandless has an interesting visual station on his site Information is Beautiful. It's sort of Venn-diagramy except instead of circles, it's ovals and they overlap in interesting ways to create this sort of flower shape, but it's in an attempt to answer the question what makes a good visualization. And so they are four of these ovals and each has their own topic. There's information, which is the data; story, which he describes as the concept; the goal or the function; and then finally, the visual form or the metaphor.
As you hover over, you can see different annotations pop up of where integrity fits in or interestingness or usefulness, beauty, and these ovals overlap with each other in different ways where you have different annotations that describe what the overlap, for example, of story and goal is or information and visual form. He has the section labeled where all of these four ovals overlap as successful visualizing. So that's one way to think about things. There would be reasons why we might be further out on one oval or another, I think, depending upon the context, depending on, as we've talked about, why we're visualizing the data, who we're visualizing it for. For me, the most important question to come back to when it comes to assessing that is what is the goal? Right, what are we trying to achieve through the visualization of data? Because if we can clearly articulate that, then we give something for someone else to assess against when we're trying to figure out is this good; right, does this do what I want it to do effectively. Because without that lens, without that context, it's hard to say when someone doesn't have visibility into the constraints that someone faces or why they're doing something in the first place.
So I don't know that it is the visualizers, going back to Charlie's question, that it's their obligation to have to say when they're doing something that may not be best practice, or if they're trying a novel approach for the sake of novelty, but I think any context that people, as they're visualizing data and especially when it's something that is in a public forum, any context they can lend to why are they doing it, what is their goal, will help everybody assess that. Because in some cases it might be oh, hey, I wanted to see if I could do this really interesting thing in this specific tool where we know it's not the best way or the most efficient way, I should say, to get the information across. But efficiency isn't the only goal, as we've talked about, in visualizing data. So I think the more that people can share about the context of why they're visualizing it, what they're hoping to get out of it, then the better we can assess what to emulate and what not and make our own conclusions when it comes to that as well.
So I want to come back just one more time to one of the components of Charlie's question, which was that today anyone can be putting data visualization out there on Twitter and get eyeballs on it, because there's another question related to what we've been talking about, which is who visualizes data. And that has changed dramatically just over the past couple of decades, because it used to be that data visualization was done by experts in a given field, often scientific fields, drawing their data by hand.
RJ Andrews has an interesting article on Florence Nightingale that was published not so long ago as part of the Data Visualization Society's medium -- which is also called Nightingale that I'll make sure to link to -- and talks about all of the different innovative sort of visualization that she was doing then and all by hand, which is very different from today where anyone can pick up a tool and make a graph, which I often describe as both fantastically awesome and super dangerous because oftentimes, we don't learn how to do this.
So it is through a lot of trial and error that we are, because today really everyone, nearly, visualizes data. There's so much data around us, we're collecting data in so many places, different organizations have desires to be data-driven, which means people in roles or parts of an organization that have historically not needed to touch data are being asked to do so and increasingly to then visualize that data as well so that we can make it meaningful and informed and drive decision making.
One common question that comes up is how should I visualize data? We will touch on that when we get back from a short break.
[COMMERCIAL] Podcast listeners we have a special for you!
Join Cole and the storytelling with data team in-person on Tuesday, March 10th in Austin Texas for a hands-on and interactive full day workshop where you’ll learn the science and art of effective data storytelling. Come see what everyone is talking about and leave with new skills that will help you drive positive change with your data stories.
For loyal podcast listeners, enter the code podcast10 to receive 10% off your registration fee. That’s podcast10 to receive 10% off your registration fee. Workshops typically sell out so don’t delay. And if you’re interested in other locations, be sure to check out storytellingwithdata.com, click attend and public workshops to see our other 2020 destinations.
We kicked off our time today thinking about what data visualization is, talked about why we visualize data, what makes it good, who visualizes data, and this idea that increasingly, many people are being asked to visualize data as part of their work. So especially for folks who are just coming into this, a common question is how do I visualize data, right; where do I start?
There are so many tools that can be used to visualize data effectively, and it doesn't take fancy tools to do this stuff well. Common ones, you have Excel, Tableau, Power BI, Flourish, Datawrapper, Google Data Studio, RD3, and that can be overwhelming. So if you're finding yourself overwhelmed by the prospect of trying to figure out what tool do I use, maybe don't start there. Start a step before that. Right, we can go back to history and put ourselves in the shoes of Florence Nightingale and just get a piece of paper and start drawing our data.
This can do really interesting things, both for freeing ourselves up from any constraints that we may have from our tools, but then just it forces you, when you're putting pen or pencil to paper, to be thoughtful about what you're doing. We can also iterate through different views really quickly.
Actually, there's an exercise in the storytelling with data community that I'll be sure to link to that asks you to do this specifically, where there's some relatively simple data posed and it's graphed so you can see it, and then you are asked to draw as many different ways as you can come up with to visualize this specific data. That practice of drawing can help us iterate through different views quickly to see what could work, what may not work. Also, as I've talked about many times before, we're less likely to form attachment to what we've done because it can be quick and dirty iterating. And what you can do then is if you can get your idea right or mostly right on paper, then you can look to say all right, what tools do I have at my disposal or what colleagues can I lean on, what expertise can I use internally or externally to try to realize those ideas?
Actually, for those who may be assessing different tools, one of the resources that we share as part of Let's Practice, my latest book, is there is a page where you can download all of the exercises and solutions for all of the exercises that are solved. There are a number of solutions that we've built out in different tools. In particular, there's one solution that shows both a line chart and a bar chart, the same one for a given solution that is created across seven different tools, which can be a nice thing, just to be able to flip through and see what's achievable in different tools and go from there.
As a final question related to this topic, let's talk about when you should visualize data. I think the answer to when should you visualize data is when seeing something is going to help build an understanding that is otherwise difficult, that encompasses a lot of things when we're thinking about working with data. We can do that. You know, going back to one of the things we talked about before and who we're doing it for, if we're doing it for ourselves, we can visualize the data when we need to explore it and try to understand where there may be something interesting going on. We can also visualize the data when we've found an interesting thing that we then need to explain to somebody else.
As a related question about when you should visualize data comes another question that underlies a lot of this, which is which data should you visualize in the first place? And even after you've landed on the data that you should visualize, do you show all of the data or some aggregation? Often it's useful to look at both and iterate through different views. Right, try looking at all of the data so that you can see the underlying distribution and then see what happens when you aggregate in different ways, whether it's different time points or by a different dimension.
Nathan Yow has a really nice example that he uses in his book Data Points where he gives a specific personal example and talks about different levels of aggregation and what you can more or less easily see and do with these different levels of aggregation. It's never the case that one is right and another is wrong, or rarely is that the case, but, rather, being really cognizant about what we gain and what we lose potentially through different views of the data. And as many things, soliciting feedback, input from other people, can be useful in helping answer many of the questions that we've talked about here today.
So those were some quick and totally incomplete, but starter thoughts related to the question what is data visualization.
What is data visualization? Turning numbers into pictures. But not just that: also being clear on your goal—why are you visualizing it and for whom are you visualizing it? By being thoughtful about the answers to these questions as you visualize data, or consume data visualizations made by others with that context in mind, we can each create and help others create better data viz.
Before we wrap, a couple of quick updates:
Our 2020 public workshop schedule has been set: you can join me, and in some instances the entire SWD team for a day of hands-on learning in London, Austin, Milwaukee, NYC, or Seattle. Info and registration at storytellingwithdata.com/public-workshops.
Speaking of workshops, we’ve added a brand new half-day workshop to our custom offerings based on my latest book, Let’s Practice! We also have a variety of webinar topics available for groups interested in learning more about effectively communicating with data. Details on all of this can be found at storytellingwithdata.com/custom-workshops.
If you’d prefer to practice on your own, be sure to check out the SWD community: your online destination for practicing, giving and receiving feedback, and discovering great work. Join today at community.storytellingwithdata.com.
For even more, you can follow on Twitter @storywithdata or check out our daily posts on LinkedIn; if you enjoy this podcast, please leave a review and share with a friend. Thanks for tuning in!
Listen to the SWD podcast on your favorite platform
Subscribe in your favorite podcast platform to never miss an episode.
Like what you hear? Please rate & review. Thanks for listening!